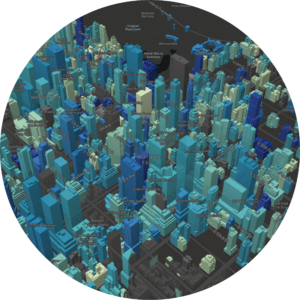
The reduction of energy use and greenhouse gas (GHG) emissions in the urban built environment has emerged as one of the greatest challenges of the 21st century. The Paris Climate Agreement calls on the global community to limit global temperature rise to 1.5 degrees Celsius through significant reductions in carbon emissions. Given the need for immediate action, cities and urban areas are increasingly taking the lead in addressing this challenge, as cities are positioned to make substantial impacts through improvements to building energy efficiency, and face dramatic consequences of inaction through increased risk from sea-level rise and extreme events.
Our aim is to advance energy and carbon modeling and public policy and investment decisions in cities in a way that is efficient, equitable, scalable, and rapidly deployable. This work, in collaboration with city, federal, and international organizations, develops computational methods and data tools that combine data-driven statistical and physical models to generate hyperlocal models of urban carbon emissions, building energy performance, and energy-use behaviors. Our focus is on understanding the disparate impacts of climate change and energy inefficiency, and developing methods to prioritize social justice in urban climate policy.
Recent projects
Building retrofit hurdle rates and risk aversion in energy efficiency investments
Despite extensive empirical evidence of the environmental benefits of green buildings and the increasing urgency to reduce carbon emissions in cities, there has been limited widespread adoption of energy retrofit investments in existing buildings. In this paper, we empirically model financial returns to energy retrofit investments for more than 3600 multifamily and commercial buildings in New York City, using a comprehensive database of energy audits and renovation work extracted from city records using a natural language processing algorithm. Based on auditor cost and savings estimates, the median internal rate of return for adopted energy conservation measures is 21% for multifamily buildings and 25% for office properties. Logistic regression modeling demonstrates adoption rates are higher for office buildings than multifamily, and in both cases adopter buildings tend to be larger, higher value, and less energy efficient prior to retrofit implementation. The economically significant magnitudes of returns to adopted energy conservation measures raise important questions about why many property owners choose not to adopt. As such, we discuss incentive and regulatory mechanisms that can overcome financial and informational barriers to the adoption of energy efficiency measures.
The impact of mandatory energy audits on building energy use
Cities are increasingly adopting energy policies that reduce information asymmetries and knowledge gaps through data transparency, including energy disclosure and mandatory audit requirements for existing buildings. Although such audits impose non-trivial costs on building owners, their energy use impacts have not been empirically evaluated. Here we examine the effect of a large-scale mandatory audit policy—New York City’s Local Law 87—on building energy use, using detailed audit and energy data between 2011 and 2016 for approximately 4,000 buildings. This specific policy context, in which the compliance year is randomly assigned, provides a unique opportunity to explore the audit effect without the self-selection bias found in studies of voluntary audit policies. We find energy use reductions of approximately –2.5% for multifamily residential buildings and –4.9% for office buildings. The results suggest that mandatory audits, by themselves, create an insufficient incentive to invest in energy efficiency at the scale needed to meet citywide carbon-reduction goals.
Inventory of New York City Green House Gas Emissions
Climate change has reached a crucial tipping point: our actions right now will determine the long-term health and even survival of our community and our planet. The City of New York has committed to reducing its greenhouse gas (GHG) emissions 80 percent by 2050, compared to 2005 levels. We have further committed to do our part to fulfill the Paris Agreement by accelerating our progress and developing strategies to achieve carbon neutrality by 2050. These strategies have the potential to remove 10 million tons of carbon dioxide equivalent from our air by 2020 and 500,000 pounds of fine particulate matter by 2030, preventing 40 deaths and 100 hospital visits every single year. In addition to fighting climate change, our actions will provide economic innovation, improved public health, and quality jobs for New Yorkers.
NYC Energy and Water Performance Map
In partnership with the New York City Mayor’s Office of Sustainability, Professor Constantine Kontokosta and his research team at NYU recently launched the NYC Energy & Water Performance Map. The website provides an interactive platform to view, query, and analyze seven years of energy, water, and carbon emissions data collected under Local Law 84 for more than 20,000 properties in New York City. Users can compare buildings by energy use, water use, greenhouse gas (GHG) intensity, type, size, age, Energy Star scores, and other metrics. Users can also see how individual buildings perform against their peers in any given year or over time. The site is searchable by address and borough-block-lot (BBL). Data from all queries can be downloaded.
The site is designed to assist the real estate industry, city agencies, and the general public in better understanding pathways to steep reductions in GHG emissions over the next 30 years. This tool is fully scalable and transferable to other cities and large portfolios.
Energy Cost Burdens for Low-Income and Minority Households
Of the three primary components of housing affordability measures—rent, transportation, and utilities—utility costs are the least understood yet are the one area where the cost burden can be reduced without household relocation. Existing data sources to estimate energy costs are limited to surveys with small samples and low spatial and temporal resolution, such as the American Housing Survey and the Residential Energy Consumption Survey. In this study, we present a new method for small-area estimates of household energy cost burdens (ECBs) that leverages actual building energy use data for approximately 13,000 multifamily properties across five U.S. cities and links energy costs to savings opportunities by analyzing 3,000 energy audit reports. We examine differentials in cost burdens across household demographic and socioeconomic characteristics and analyze spatial, regional, and building-level variations in energy use and expenditures. Our results show the average low-income household has an ECB of 7%, whereas higher income households have an average burden of 2%. Notably, even within defined income bands, minority households experience higher ECBs than non-Hispanic White households. For lower income households, low-cost energy improvements could reduce energy costs by as much as $1,500 per year.
In this study we attempt to shift the focus of energy efficiency investments to their impact on household cost burdens and overall housing affordability. Our analysis explores new and unique data generated from measurement-driven urban energy policies and shows low-income households disproportionately bear the burden of poor-quality and energy-inefficient housing. Cities can use these new data resources and methods to develop equity-based energy policies that treat energy efficiency and climate mitigation as issues of environmental justice and that apply data-driven, targeted policies to improve quality of life for the most vulnerable urban residents.
Grading buildings on energy performance using city benchmarking data
As the effects of anthropogenic climate change become more pronounced, local and federal governments are turning towards more aggressive policies to reduce energy use in existing buildings, a major global contributor of carbon emissions. Recently, several cities have enacted laws mandating owners of large buildings to publicly display an energy efficiency rating for their properties. While such transparency is necessary for market-driven energy reduction policies, the reliance on public-facing energy efficiency grades raises non-trivial questions about the robustness and reliability of methods used to measure and benchmark the energy performance of existing buildings. In this paper, we develop a building energy performance grading methodology using machine learning and city-specific energy use and building data. Leveraging the growing availability of data from city energy disclosure ordinances, we develop the GREEN grading system: a framework to facilitate more accurate, fair, and contextualized building energy benchmarks that account for variations in the expected and actual performance of individual buildings. When applied to approximately 7500 residential properties in New York City, our approach accounts for the differential impact of design, occupancy, use, and systems on energy performance, out-performing existing state-of-the-art methods. Our model and findings reinforce the need for more robust, localized approaches to building energy performance grading that can serve as the basis for data-driven urban energy efficiency and carbon reeduction policies.