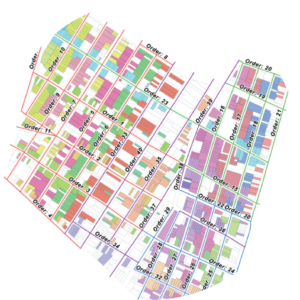
Analytics and data-driven decision-making represent a significant opportunity to revolutionize the efficient and equitable delivery of city services and the design and implementation of policy interventions. As resources tighten and demands for city services increase, the application of improved data collection methods, new analytical tools, and better operational intelligence that yields actionable insight is becoming the foundation for next-generation city management and planning practice. Our work in this area couples computational methods with data of diverse types, structures, and sources to address a range of applied problems in city operations.
Recent projects
Up-and-Coming or Down-and-Out? Social Media Popularity as an Indicator of Neighborhood Change
By quantifying Twitter activity and sentiment for each of 274 neighborhood areas in New York City, this study introduces the Neighborhood Popularity Index and correlates changes in the index with real estate prices, a common measure of neighborhood change. Results show that social media provide both a near-real-time indicator of shifting attitudes toward neighborhoods and an early warning measure of future changes in neighborhood composition and demand. Although social media data provide an important complement to traditional data sources, the use of social media for neighborhood studies raises concerns regarding data accessibility and equity issues in data representativeness and bias.
Take the Q Train: Value Capture of Public Infrastructure Projects
Topic modeling to discover the thematic structure and spatial-temporal patterns of building renovation and adaptive reuse in cities
Building alteration and redevelopment play a central role in the revitalization of developed cities, where the scarcity of available land limits the construction of new buildings. The adaptive reuse of existing space reflects the underlying socioeconomic dynamics of the city and can be a leading indicator of economic growth and diversification. However, the collective understanding of building alteration patterns is constrained by significant barriers to data accessibility and analysis. We present a data mining and knowledge discovery process for extracting, analyzing, and integrating building permit data for more than 2,500,000 alteration projects from seven major U.S. cities. We utilize natural
language processing and topic modeling to discover the thematic structure of construction activities from permit descriptions and merge with other urban data to explore the dynamics of urban change. The knowledge discovery process proceeds in three steps: (1) text mining to identify popular words, popularity change, and their co-appearance likelihood; (2) topic modeling using latent Dirichlet allocation (LDA); and (3) integrating the topic modeling output with building information and ancillary data to discover the spatial, temporal, and thematic patterns of urban redevelopment and regeneration. The results demonstrate a generalizable approach that can be used to analyze unstructured text data extracted from permit records across varying database structures, permit typologies, and local contexts. Our machine learning methodology can assist cities to better monitor building alteration activity, analyze spatiotemporal patterns of redevelopment, and more fully understand the economic, social, and environmental implications of changes to the urban built environment.