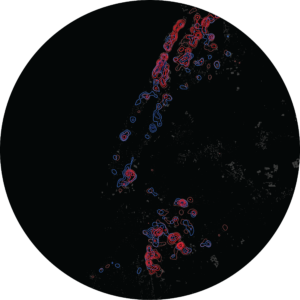
Predictive algorithms carry systemic biases resulting from persistent spatial, racial, and economic inequalities in cities. Decisions based on machine learning models, particularly those trained on resident-reported complaint data, can result in discriminatory outcomes and a mis-allocation of city resources, further reinforcing disparities in neighborhood quality. Our work on bias and fairness in data-driven decision-making addresses these issues in the urban context. Our research involves (1) identifying and measuring reporting biases; (2) providing analytical tools to city decision-makers, policymakers, and planners to understand and visualize the spatial and socioeconomic dependence of reporting behaviors; and (3) developing methods to account for observed biases in responding to resident reports. The focus of this work is currently on ‘311’ complaint data.
Recent projects
Bias in smart city governance: How socio-spatial disparities in 311 complaint behavior impact the fairness of data-driven decisions
Governance and decision-making in “smart” cities increasingly rely on resident-reported data and data-driven methods to improve the efficiency of city operations and planning. However, the issue of bias in these data and the fairness of outcomes in smart cities has received relatively limited attention. This is a troubling and significant omission, as social equity should be a critical aspect of smart cities and needs to be addressed and accounted for in the use of new technologies and data tools. This paper examines bias in resident-reported data by analyzing socio-spatial disparities in ‘311’ complaint behavior in Kansas City, Missouri. We utilize data from detailed 311 reports and a comprehensive resident satisfaction survey, and spatially join these data with code enforcement violations, neighborhood characteristics, and street condition assessments. We introduce a model to identify disparities in resident-government interactions and classify under- and over-reporting neighborhoods based on complaint behavior. Despite greater objective and subjective need, low-income and minority neighborhoods are less likely to report street condition or “nuisance” issues, while prioritizing more serious problems. Our findings form the basis for acknowledging and accounting for data bias in self-reported data, and contribute to the more equitable delivery of city services through bias-aware data-driven processes.
Equity in 311 Reporting: Understanding Socio-Spatial Differentials in the Propensity to Complain
Cities across the United States are implementing information communication technologies in an effort to improve government services. One such innovation in e-government is the creation of 311 systems, offering a centralized platform where citizens can request services, report non-emergency concerns, and obtain information about the city via hotline, mobile, or web-based applications. The NYC 311 service request system represents one of the most significant links between citizens and city government, accounting for more than 8,000,000 requests annually. These systems are generating massive amounts of data that, when properly managed, cleaned, and mined, can yield significant insights into the real-time condition of the city. Increasingly, these data are being used to develop predictive models of citizen concerns and problem conditions within the city. However, predictive models trained on these data can suffer from biases in the propensity to make a request that can vary based on socio-economic and demographic characteristics of an area, cultural differences that can affect citizens’ willingness to interact with their government, and differential access to Internet connectivity. Using more than 20,000,000 311 requests – together with building violation data from the NYC Department of Buildings and the NYC Department of Housing Preservation and Development; property data from NYC Department of City Planning; and demographic and socioeconomic data from the U.S. Census American Community Survey – we develop a two-step methodology to evaluate the propensity to complain: (1) we predict, using a gradient boosting regression model, the likelihood of heating and hot water violations for a given building, and (2) we then compare the actual complaint volume for buildings with predicted violations to quantify discrepancies across the City.
NYC311
Joe Morrisroe, executive director for NYC311, had some gut instincts but no definitive answer to the question he was just asked by one of the mayor’s deputies: “Are some communities being underserved by 311? How do we know we are hearing from the right people?” Founded in 2003 as a phone number for residents to dial (311) from a landline for information on city services and to log complaints, the city launched a 311 website and mobile app in 2009 and social media support in 2011. In 2016, NYC311 received over 35 million requests for services and information. Technological progress had made it considerably easier to hear from NYC residents. Were those gains from innovation being shared equally? More recently, the city began using the data to create predictive models that might help direct inspectors and other workers. Morrisroe and his team had considered the potential downsides of agencies relying too heavily on NYC311 data or on its predictive power. In the sheer volume of the data and its potential to enable a new approach to city services, were biases around income, education, race, gender, neighborhood, home ownership, and other factors hiding too? Morrisroe considered the question posed to him and its implications. He asked for the data and a team to assess it: Are we hearing from everyone?